Model Design using Pytorch
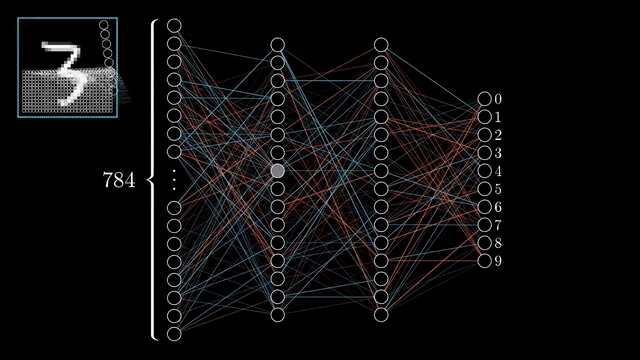
Source: 3Blue1Brown
Neural Network has been evolving recently. Many applications have been developed by using neural network. The development of GPU provided by big company like NVIDIA has fasten how the training process in Architecture of Neural network that needs many parameters in order to get better result for any kind of problems. You can see on the GIf above how neural network works throguh many layers that involve many parameters that can create good output that can identify the real value. The practical way is image identification where Neural network through combining many layers and parameters, activation function, and loss that can be improved to identify the image based on the GIF above. We will learn the implementation through Pytorch in this tutorial.
import pandas as pd
import numpy as np
import matplotlib.pyplot as plt
import torch
import torch.nn as nn
import torch.optim as optim
import warnings
warnings.filterwarnings("ignore")
df = pd.read_csv("SomervilleHappinessSurvey2015.csv")
df.head()
Columns Information:
- D = decision attribute (D) with values 0 (unhappy) and 1 (happy)
- X1 = the availability of information about the city services
- X2 = the cost of housing
- X3 = the overall quality of public schools
- X4 = your trust in the local police
- X5 = the maintenance of streets and sidewalks
-
X6 = the availability of social community events
-
Attributes X1 to X6 have values 1 to 5.
X = torch.tensor(df.drop("D",axis=1).astype(np.float32).values)
y = torch.tensor(df["D"].astype(np.float32).values)
X[:10]
# 6 from how many features we have
# output whether happy or unhappy
model = nn.Sequential(nn.Linear(6,1),
nn.Sigmoid())
print(model)
loss_func = nn.MSELoss()
optimizer = optim.Adam(model.parameters(),lr=1e-2)
losses =[]
for i in range(20):
y_pred = model(X)
loss = loss_func(y_pred,y)
# item() is used for getting value from tensor
losses.append(loss.item())
optimizer.zero_grad()
#do back propagation
loss.backward()
#update weights during backward propagation
optimizer.step()
if i%5 ==0:
print(i,loss.item())
plt.plot(range(0,20),losses)
plt.xlabel("Epoch")
plt.ylabel("Loss")
This is just a simple sequential neural network by implementing pytorch. We will deep dive into a process of building model in the study case 2 where we implement the process of Data Science lifecycle from cleaning the data,splitting the data, making the prediction and evaluating the prediction.
Deep Learning in Bank
Deep Learning has been implementing in many sectors including Bank.The problem thas has been happening for this sector is to predict whether bank should grant loan for the customers who will be making credit card. This is essential for Bank because it can measure how they can validate how much money that they can provide and estimate the profit from customers who will use the credit card based on a period of time. We will detect the customers who will be potential to grant loan that can affect to the income of the bank through this dataset.
We will follow a few steps before modelling our data into ANN using Pytorch including :
- Understand the data including dealing with quality of data
- rescale the features (giving different scales for each features may result that a given features is more important thatn others as it has higher numerical values)
- split the data
df_credit = pd.read_excel("default of credit card clients.xls",skiprows=1)
df_credit.head()
print(f"Rows : {df_credit.shape[0]}, Columns:{df_credit.shape[1]}")
data_clean =df_credit.drop(["ID","SEX"],axis=1)
data_clean.head()
(data_clean.isnull().sum()/data_clean.shape[0]).plot()
data_clean.describe()
outliers ={}
for i in range(data_clean.shape[1]):
min_t = data_clean[data_clean.columns[i]].mean()-(3*data_clean[data_clean.columns[i]].std())
max_t = data_clean[data_clean.columns[i]].mean()+(3*data_clean[data_clean.columns[i]].std())
count =0
for j in data_clean[data_clean.columns[i]]:
if j < min_t or j > max_t:
count +=1
percentage = count/data_clean.shape[0]
outliers[data_clean.columns[i]] = round(percentage,3)
from pprint import pprint
pprint(outliers)
data_clean["default payment next month"].value_counts().plot(kind="bar")
target = data_clean["default payment next month"]
yes = target[target == 1].count()
no = target[target == 0].count()
data_yes = data_clean[data_clean["default payment next month"] == 1]
data_no = data_clean[data_clean["default payment next month"] == 0]
over_sampling = data_yes.sample(no, replace=True, \
random_state = 0)
data_resampled = pd.concat([data_no, over_sampling], \
axis=0)
data_resampled["default payment next month"].value_counts().plot(kind="bar")
data_resampled = data_resampled.reset_index(drop=True)
X = data_resampled.drop("default payment next month",axis=1)
y =data_resampled["default payment next month"]
X = (X-X.min())/(X.max()-X.min())
X.head()
final_data =pd.concat([X,y],axis=1)
final_data.to_csv("data_prepared.csv",index=False)
import torch.nn.functional as F
from sklearn.model_selection import train_test_split
from sklearn.utils import shuffle
from sklearn.metrics import accuracy_score
data = pd.read_csv("data_prepared.csv")
data.head()
X = data.drop("default payment next month",axis=1)
y =data["default payment next month"]
X_new , X_test,y_new,y_test =train_test_split(X,y,test_size=0.2,random_state=3)
dev_per = X_test.shape[0]/X_new.shape[0]
X_train,X_dev,y_train,y_dev = train_test_split(X_new,y_new,test_size=dev_per,random_state=3)
print("Training sets:",X_train.shape, y_train.shape)
print("Validation sets:",X_dev.shape, y_dev.shape)
print("Testing sets:",X_test.shape, y_test.shape)
X_dev_torch = torch.tensor(X_dev.values).float()
y_dev_torch = torch.tensor(y_dev.values)
X_test_torch = torch.tensor(X_test.values).float()
y_test_torch = torch.tensor(y_test.values)
class Classifier(nn.Module):
def __init__(self, input_size):
super().__init__()
self.hidden_1 = nn.Linear(input_size, 10)
self.hidden_2 = nn.Linear(10, 10)
self.hidden_3 = nn.Linear(10, 10)
self.output = nn.Linear(10, 2)
def forward(self, x):
z = F.relu(self.hidden_1(x))
z = F.relu(self.hidden_2(z))
z = F.relu(self.hidden_3(z))
out = F.log_softmax(self.output(z), dim=1)
return out
model = Classifier(X_train.shape[1])
criterion = nn.NLLLoss()
optimizer = optim.Adam(model.parameters(), lr=0.001)
epochs = 50
batch_size = 128 # for faster training procces/mini batch gradient descent
train_losses,dev_losses,train_acc,dev_acc =[],[],[],[]
for epoch in range(epochs):
X_,y_ =shuffle(X_train,y_train)
running_loss=0
running_acc=0
iterations =0
for i in range(0,len(X_),batch_size):
iterations +=1
b = i +batch_size
X_batch = torch.tensor(X_.iloc[i:b,:].values).float()
y_batch = torch.tensor(y_.iloc[i:b].values)
pred = model(X_batch)
loss = criterion(pred,y_batch)
optimizer.zero_grad()
loss.backward()
optimizer.step()
running_loss +=loss.item()
ps = torch.exp(pred)
top_p,top_class = ps.topk(1,dim=1)
running_acc +=accuracy_score(y_batch,top_class)
dev_loss =0
acc =0
with torch.no_grad():
pred_dev = model(X_dev_torch)
dev_loss =criterion(pred_dev,y_dev_torch)
ps_dev = torch.exp(pred_dev)
top_p,top_class_dev = ps_dev.topk(1,dim=1)
acc +=accuracy_score(y_dev_torch,top_class_dev)
train_losses.append(running_loss/iterations)
dev_losses.append(dev_loss)
train_acc.append(running_acc/iterations)
dev_acc.append(acc)
print("Epoch: {}/{}.. ".format(epoch+1, epochs),
"Training Loss: {:.3f}.. ".format(running_loss/iterations),
"Validation Loss: {:.3f}.. ".format(dev_loss),
"Training Accuracy: {:.3f}.. ".format(running_acc/iterations),
"Validation Accuracy: {:.3f}".format(acc))
fig = plt.subplots(figsize=(15,5))
plt.plot(train_losses,label="Training loss")
plt.plot(dev_losses,label="Validation loss")
plt.legend(frameon=False, fontsize=15)
fig = plt.subplots(figsize=(15,5))
plt.plot(train_acc,label="Training accuracy")
plt.plot(dev_acc,label="Validation accuracy")
plt.legend(frameon=False, fontsize=15)
We can see there is a change in the loss and accuracy accuracy during each epoch. We can do tune the learning rate for getting better result. You can do the experimentation by comparing the LR as well. This is a few steps in modelling using pytorch. You can see that we can do modelling by using Sequential or custom models using torch.nn.
# by adding hidden layer or epochs for training process
class Classifier_Layer(nn.Module):
def __init__(self, input_size):
super().__init__()
self.hidden_1 = nn.Linear(input_size, 10)
self.hidden_2 = nn.Linear(10, 10)
self.hidden_3 = nn.Linear(10, 10)
self.hidden_4 = nn.Linear(10, 10)
self.output = nn.Linear(10, 2)
def forward(self, x):
z = F.relu(self.hidden_1(x))
z = F.relu(self.hidden_2(z))
z = F.relu(self.hidden_3(z))
z = F.relu(self.hidden_4(z))
out = F.log_softmax(self.output(z), dim=1)
return out
model = Classifier_Layer(X_train.shape[1])
criterion = nn.NLLLoss()
optimizer = optim.Adam(model.parameters(), lr=0.01)
epochs = 100
batch_size = 150 # for faster training procces/mini batch gradient descent
train_losses,dev_losses,train_acc,dev_acc =[],[],[],[]
for epoch in range(epochs):
X_,y_ =shuffle(X_train,y_train)
running_loss=0
running_acc=0
iterations =0
for i in range(0,len(X_),batch_size):
iterations +=1
b = i +batch_size
X_batch = torch.tensor(X_.iloc[i:b,:].values).float()
y_batch = torch.tensor(y_.iloc[i:b].values)
pred = model(X_batch)
loss = criterion(pred,y_batch)
optimizer.zero_grad()
loss.backward()
optimizer.step()
running_loss +=loss.item()
ps = torch.exp(pred)
top_p,top_class = ps.topk(1,dim=1)
running_acc +=accuracy_score(y_batch,top_class)
dev_loss =0
acc =0
with torch.no_grad():
pred_dev = model(X_dev_torch)
dev_loss =criterion(pred_dev,y_dev_torch)
ps_dev = torch.exp(pred_dev)
top_p,top_class_dev = ps_dev.topk(1,dim=1)
acc +=accuracy_score(y_dev_torch,top_class_dev)
train_losses.append(running_loss/iterations)
dev_losses.append(dev_loss)
train_acc.append(running_acc/iterations)
dev_acc.append(acc)
print("Epoch: {}/{}.. ".format(epoch+1, epochs),
"Training Loss: {:.3f}.. ".format(running_loss/iterations),
"Validation Loss: {:.3f}.. ".format(dev_loss),
"Training Accuracy: {:.3f}.. ".format(running_acc/iterations),
"Validation Accuracy: {:.3f}".format(acc))
fig = plt.subplots(figsize=(15,5))
plt.plot(train_losses,label="Training loss")
plt.plot(dev_losses,label="Validation loss")
plt.legend(frameon=False, fontsize=15)
fig = plt.subplots(figsize=(15,5))
plt.plot(train_acc,label="Training accuracy")
plt.plot(dev_acc,label="Validation accuracy")
plt.legend(frameon=False, fontsize=15)
You can experiment by changing the architecture of the model